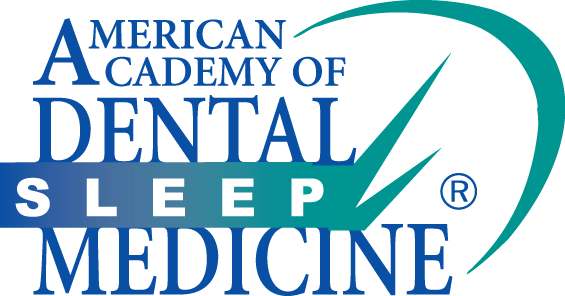
Original Article 3, Issue 2.4
Development of a Simplified Pediatric Obstructive Sleep Apnea (OSA) Screening Tool
http://dx.doi.org/10.15331/jdsm.5118
Harmeet K. Chiang, DDS, MS1 ; Jo K. Cronly, DDS, MSD2 ; Al M. Best, PhD1 ; Tegwyn H. Brickhouse, DDS, PhD1 ; David J. Leszczyszyn, MD, PhD3
1School of Dentistry, Virginia Commonwealth University, Richmond, VA; 2Private Practice, Richmond VA; 3School of Medicine, Virginia Commonwealth University, Richmond, VA
ABSTRACT
Study Objectives:
To develop and test a pediatric screening tool to gauge the risk that an individual child would have OSA prior to a dental procedure by a pediatric dentist requiring minimal or moderate oral conscious sedation.
Methods:
180 pediatric patients completed a polysomnogram at the VCU Center for Sleep Medicine between February 2011 and February 2013. A modified STOP-Bang questionnaire was validated with polysomnography.
Results:
A validated adult questionnaire, STOP-Bang, was modified using more typical pediatric risk factors for OSA: presence of snoring (S), tonsillar hypertrophy (T), obstruction (O), daytime tiredness or neuropsychological-behavioral symptoms such as ADHD or daytime irritability (P), BMI percentile for age (B), age at diagnostic screening (A), presence of neuromuscular disorder (N), and presence of genetic/congenital disorder (G). A positive scoring from these variables was measured against the patients acquired in-laboratory polysomnogram using the standard OSA measure, apnea-hypopnea index. A multiple logistic regression analysis found a statistically significant relationship (p = 0.0007), with a minimum of 4 variables needed to have a sensitivity of 57% and a specificity of 78%. Only obstruction, BMI, and age showed a strong significant relationship to OSA. The presence of an obstruction was positively related to apnea (p = 0.0010). Most of the other components had an odds ratio larger than one (indicating a nominally positive relationship).
Conclusions:
The pediatric modified STOP-Bang screening tools showed a statistically significant relationship. Only obstruction, BMI, and age showed a predictive relationship to OSA. Although the PM-STOP-Bang results do not lend support to including other known risk factors of pediatric OSA, further studies are warranted of a revised screening tool that include recognized risk factors.
Keywords:
pediatric obstructive sleep apnea, simplified screening tool
Citation:
Chiang HK, Cronly JK, Best AM, Brickhouse TH, Leszczyszyn DJ. Development of a simplified pediatric obstructive sleep apnea (OSA) screening tool. Journal of Dental Sleep Medicine 2015;2(4):163–173.
Sleep disordered breathing encompasses a wide range of upper airway disorders from primary snoring (PS) to obstructive sleep apnea (OSA). OSA results from impedance to airflow in the upper airway during sleep; these periodic obstructions of the upper airway interfere with normal respiratory gas exchange and subsequently interrupt sleep.1,2 OSA has become recognized as one of the most common, underdiagnosed chronic diseases.3–5 People of all ages are affected with OSA. Recently studies have shown increased numbers among pediatric and adolescent populations.6 The prevalence of obstructive sleep apnea (OSA) in children is estimated to be 1% to 3%,7 while primary snoring occurs in 3% to 12% of the pediatric population.8 Mild cases of pediatric OSA are recognized and at times treated; however, measurable effects on development, cardiopulmonary, or metabolic systems have been difficult to validate. OSA is associated with behavioral problems, poor school achievement, and, in severe cases, pulmonary hypertension.2 Many studies have been conducted to identify adverse effects of sleep disorders, yet few studies have examined how health care providers may identify and treat sleep disorders.10
Dentists see their patients more frequently than their primary care doctors, and so have a greater opportunity to observe signs and symptoms of OSA.6 However, many potential sleep disorders in children are unrecognized and underreported, and overall the condition is under-diagnosed.11 Dentists who practice sedation dentistry should exercise extra precautions when treating patients with risk of sleep apnea. Minimal and moderate oral conscious sedation and general anesthesia are commonly used in pediatric dentistry. During sedation, children with OSA have an increased vulnerability of their airway undergoing pharyngeal collapse and of having upper airway obstruction.7 Thus pediatric dentists have an acute responsibility to be able to identify patients who may have OSA.5 The risk of postoperative respiratory complications among the pediatric population ranges from 0 to 1.3%; however, for children with OSA, the rates have been reported to be 16% to 27%.12,13 The prevalence of OSA in children is most elevated between 2 to 6 years of age. In this age range, pharmacologic measures are most often used to complete diagnostic and therapeutic procedures.7
While polysomnography (PSG) remains the gold standard for diagnosing OSA, there are many challenges due to the limited number of sleep laboratories and the high cost of performing a PSG on each child who snores and who may be at risk.8 Available non-PSG screening tests have poor sensitivity for milder OSA, and overall poor specificity.8 Moreover, there remains a challenge to differentiate PS from OSA in a “cost-effective, reliable, and accurate manner before recommending invasive or intrusive therapies, such as surgery or continuous positive airway pressure.”8
Sleep questionnaires that are completed by the parent and child are a crucial component of behavioral and physiological sleep assessment. Pediatric questionnaires are mostly retrospective in that the parents report on past sleep patterns and behaviors that are typical of their child. In 2008, Chung et al. developed and validated a STOP questionnaire as a screening tool for OSA in patients 18 years and older. This questionnaire asks four yes/no questions: do you snore loudly?, do you feel tired during the daytime?, has anyone observed you stop breathing during your sleep?, and do you have high blood pressure? These questions along with body mass index, age, neck size, and gender (BANG) were found to have a sensitivity of 83.6, 92.9, and 100% (for mild, moderate, and severe OSA, respectively).15 In 2006, the American Society of Anesthesiologists (ASA) Task Force on Perioperative Management of Patients with Obstructive Sleep Apnea recommended a checklist as a routine screening tool to utilize in surgical patients who may have OSA. The ASA checklist has 12 items for adults and 14 items for children, but was only validated by Chung for its use on adults.16
In 2011 Spruyt and Gozal published a review on pediatric sleep questionnaires that examined 57 sleep measures that were used to screen children for sleep disorders including OSA.14 Only 2 questionnaires fulfilled all desirable criteria: The Sleep Disturbance Scale for Children (SDSC) at a cutoff score of 39 provided a sensitivity of 0.89 and a specificity of 0.74; and The Sleep Disorders Inventory for Students-Children (SDIS-C) showed a sensitivity of 0.91 and a specificity of 0.62 for the category of obstructive sleep apnea syndrome.17,18 This review documented that few standardized screening tools exist thus far to determine risk for OSA in children.14 Pediatric dentists and anesthesiologists alike would benefit from a standard screening tool, similar to the STOP-Bang, to determine if OSA may exist in potential sedation and anesthesia pediatric patients.
OSA is often the Achilles heel of pediatric sedation and analgesic programs7 ; thus it is imperative that pediatricians and pediatric dentists be able to identify a child who may be at risk for OSA so that appropriate referrals for a definitive diagnosis can be made. Currently there is no screening tool available to pediatric dentists to aid in recognizing OSA during the preoperative appointment or to help direct specialty consultation for patients undergoing minimal and moderate oral conscious sedation.
The primary aim of the study was to modify the STOP-Bang instrument for use in a pediatric setting and test the scale to screen for sleep apnea in children. The secondary aims were to test each of the components of the instrument.
METHODS
This project was granted an exempt status from the Virginia Commonwealth University Institutional Review Board (# HM15027). This was a retrospective chart review of the routine exam of patients referred for a sleep study. The original data was collected at the Center for Sleep Medicine for pediatric patients (under age 18) from February 1, 2011, to February 1, 2013, with no previous sleep disorder diagnosis. Study data were collected and managed using REDCap.20 To be included, patients had to have a completed polysomnogram and a completed sleep questionnaire (Appendix 2) in the chart record. Using data from the Medical College of Virginia electronic health record and the Sleep Center database, 180 patients were eligible. The following variables were collected: age of patient at time of PSG, gender, race, height, weight, body mass index, presence of snoring, presence of tonsillar hypertrophy, obstruction while sleeping, presence of neurobehavioral symptoms, daytime tiredness or irritability, presence of neuromuscular disorders, presence of genetic disorders, and apnea-hypopnea index (AHI). The child’s age, gender, height and weight was used to verify the reported BMI and to calculate the BMI-percentile-for-age (using the nccd.cdc.gov/dnpabmi/ Calculator.aspx calculator). The presence of snoring, obstruction while sleeping, and daytime tiredness or irritability was determined by the sleep study questionnaire completed by the parent and patient prior to the PSG.
Apnea-Hypopnea Index (AHI)
OSA was diagnosed by a patient’s apnea-hypopnea index (AHI). The AHI represents the average number of apneas and hypopneas per hour of sleep.8 In pediatric OSA, which has the same prevalence in boys and girls, more than one obstructive apnea event of any length per hour of sleep is considered abnormal.21,22,23 Based on these recommendations, apnea was categorized as: none (AHI ≤ 1.5), mild (AHI > 1.5), moderate (AHI > 5), or severe (AHI > 15). The primary categorization was a binary outcome: apnea negative (AHI ≤ 5) or apnea positive (AHI > 5).
Pediatric Modified (PM) STOP-Bang
PM-STOP-Bang (heretofore simply referred to as STOP-Bang) was the sum of the presence of snoring (S), tonsillar hypertrophy (T), observed obstruction (O), neuropsychological-behavioral symptoms such as ADHD or daytime irritability (P), BMI percentile for age and gender above 95% (B), age at diagnostic screening (A), presence of neuromuscular disorder (N), and presence of genetic/congenital disorder (G). Yes values were scored as 1 and all other values (No and unknown) were scored as zero.Data Analysis
All analyses were performed using SAS software (JMP pro version 11, SAS version 9.3, SAS Institute Inc., Cary NC). The statistical methods included screening of each diagnostic characteristic (using χ2 analysis) and a multiple logistic regression analysis of the OSA diagnosis to determine which diagnostics characteristics are associated with the diagnosis. Final reporting included odds ratios and 95% confidence intervals on all estimates.Using the projected 250 charts that were initially thought to be available, and estimating the prevalence OSA ≥ 25% and odds ratios ≥ 2, the study had approximately 80% power (at α = 0.05).
RESULTS
After excluding 27 because of incomplete data, 153 subjects with usable data were analyzed (see Table 1). Neither gender (p = 0.4455) nor race (p = 0.1368) appeared related to the AHI scores. Subjects ranged in age from 38 months to 17.5 years. The average BMI percentile for age was 73%. There were 60 subjects (39%) who were described as obese since they were above the 95th percentile for age and gender.
Table 1Demographic characteristics of study subjects (n = 153). |
AHI
The primary outcome variable was observed apneas and hypopneas, as indicated by AHI. The raw AHI values ranged from 0 to 85.7 apnea-hypopnea events per hour, with a median value of 0.8. The strongly skewed values yielded a mean of 4.08 (SD 9.53). There were 82% considered negative for apnea (96 = none, and 29 = mild), and therefore 18% (16 moderate and 12 severe) were considered positive.Scale Values
The components of the STOP-Bang scale are summarized in the prevalence column of Table 2. For instance, over 59% of all subjects had a positive indication in the medical record for snoring (n = 91). Thus the prevalence of each of these components ranged from a high of 60% (psychological symptoms) to a low of 14% for both neuromuscular disorders and for genetic/ congenital disorders.
Table 2Components of STOP-Bang and the relationship with obstructive sleep apnea. |
For the STOP-Bang scale the scores ranged from 0 to 6 (Mean = 2.76, SD = 1.34). Logistic regression was used to test for a relationship between the STOP-Bang scale and apnea. There was a statistically significant relationship (likelihood ratio χ2 = 11.5, p = 0.0007). The stacked bar chart in Figure 1 shows that for STOP-Bang scores ≤ 3, at least 72% were categorized as OSA = none (the white area of the graph), and that this white proportion decreases with increasing STOP-Bang. And as STOP-Bang increases the proportion of patients with OSA = severe (red) and the proportion with OSA = moderate (black) is < 11% for those with STOP-Bang scores ≤ 3, and it increases to 20% OSA positive for STOPBang = 4 and 58% positive for STOP-Bang = 5 or 6.
Figure 1Results for PM-STOP-Bang. |
Table 3 shows the relationship between each scale value and the sensitivity and specificity. For instance, if STOP-Bang ≥ 6 is used as a cutoff, then 2 subjects were predicted to be positive. Of the 28 actual positives, one had a cutoff ≥ 6 and so the sensitivity was 4% (1/28). Of the 125 actual negatives, all but one had a cutoff < 6, so the specificity was 99% (124/125). If the risk of false positives and false negatives were equal, then the cutoff yielding the largest sensitivity + specificity would be the optimal cutoff. For a cutoff of STOP-Bang ≥ 4, sensitivity was 57% and specificity was 78%.
Table 3STOP-Bang scale results. |
Analysis of the Components
Each of the individual components was first screened using an unadjusted χ2 test (Table 2). There were 91 patients with a snoring risk indicator, and 21 of them (23%) were positive for OSA. This is compared to 11% positive for OSA in the group of 62 patients without a snoring risk indicator. Although the odds ratio was large (2.36), it was not statistically significant (p = 0.064). The results indicate that the only statistically significant risk factor was sleep obstruction (p = 0.001). However all components had a relative risk value > 1 except for neuropsychological-behavioral symptoms/tiredness (OR = 0.72). A multiple logistic regression analysis was used to test the significance of each of the components of the scales and shows the results for the components of STOP-Bang (adjusted columns in Table 2). Although the test that all 8 components provided predictive value was significant (p = 0.0024), only one component was individually significant. The presence of an obstruction was positively related to apnea (p = 0.001). Most of the other components had an odds ratio larger > 1 (indicating a nominally positive relationship). However, two components— snoring and neurobehavioral symptoms/daytime tiredness had odds ratios < 1, which indicates that the presence of the component is negatively related to apnea.DISCUSSION
In this retrospective chart review, specific variables were compared with AHI scores in order to develop a screening tool with a high sensitivity and specificity for pediatric obstructive sleep apnea. The literature indicates that less than half of children with OSA symptoms actually have the syndrome.24 As a result, screening for OSA is challenging and causes many children to go undiagnosed. Presently, pediatric OSA is under-diagnosed and thus undertreated because of the high cost to test for OSA and the limited number of pediatric sleep laboratories.
Consequently screening for OSA has become essential.25 Canto’s recent systematic review and meta-analysis explored the diagnostic value of alternative methods such as clinical history and physical examination to identify pediatric OSA, and also validated the role dentists play in screening patients.26 In the following discussion, the findings of the current study will be compared to the results of Canto’s systematic review where applicable. The results of the current study found a clinically significant correlation between the proposed STOP-Bang scale and AHI. However, only one individual component was strongly related to AHI. This suggests that certain variables that present together in a single individual may predispose that person to OSA more than individual parameters.
Below each variable evaluated in this study is dissected along with present findings and suggestions for a revised screening tool based on these results.
STOP-Bang: Snoring is the first component of both the adult STOP-Bang and the PM-STOP-Bang. In a review on sleep disordered breathing in children, Padmanabhan et al. ascertained that snoring, apnea, and difficulty in breathing were the three main symptoms of OSA in children and infants. Snoring occurs in almost all children with a sleep disorder; often it is the catalyst for parents to believe there is a problem and to pursue a medical evaluation.27 Furthermore, snoring remains the most common complaint in sleep disordered breathing for children under five years old.21 However, only a fraction of children who snore have OSA,27,28 and the presence of snoring alone cannot accurately predict OSA.8 The correlation between snoring and AHI in our study overall had a weak relationship both individually (p > 0.06), and once all values were adjusted for, it became nonsignificant (p > 0.6). Thus, the presence of snoring does not automatically indicate that the child has OSA.8 Young et al. determined that 10% to 14% of children snore at least every other night, and found a prevalence of OSA in 10% to 20% of habitual snorers.29 Our results are similar. One limitation of our study was that snoring was not uniformly described in the medical charts, and so parents may have reported their child snored even when it was infrequent. Also problematic is that parents likely have varying subjective standards for what they consider “snoring” and also vary in their opportunity to observe the behavior. Snoring alone is not a sensitive indicator of OSA, but because it is a prevalent symptom of OSA it remains a useful variable in our screening tool.
STOP-Bang: We used the presence of tonsillar hypertrophy for the T component of our modified STOP-Bang but it did not show a significant correlation with AHI (p > 0.2). However, the most common identified risk factor in childhood OSA is adenotonsillar hypertrophy.2,31,32 The primary treatment for OSA in children is adenotonsillectomy.2,31,32 In Marcus’ Childhood Adenotonsillectomy Trial (CHAT), a watchful waiting group was compared to early removal of the tonsils in school-age children. This study found that patient’s symptoms overall improved as well as quality of life and polysomnography findings. However surgical treatment did not improve attention or function evaluated through neuropsychological testing.31 Like snoring, the presence of large tonsils does not necessarily result in OSA. Several studies have reported that no relationship exists between the size of the tonsil and adenoids and the presence of OSA.8,33,34 Canto’s systematic review found overall weak results concerning tonsils: with sensitivity = 69% and specificity = 53% for tonsillar hypertrophy and sensitivity = 81% and specificity = 58% for Grade 3 tonsil size.26 Although our study found no relationship with the size of tonsils, it was not always reliably recorded. In this study a little less than half (72 of 153) of the subjects’ tonsil size was unable to be determined. These unknowns were recorded as “no” in the data analysis. These limitations likely cause the data in this study to underreport tonsillar hypertrophy. Despite these results and the lack of literature ascertaining tonsillar hypertrophy to predict OSA, it continues to a major cause of OSA2 and consequently will remain in the revised screening tool.
STOP-Bang: Sleep obstruction is another common symptom of children with OSA and represented the O in our study. Obstructive apnea occurs when there is respiratory effort and lack of airflow.30 Our results exemplified a strong ordinal relationship with AHI and obstruction (p = 0.0010), with a sensitivity of 71% and a specificity of 69%. There is a strong correlation for two reasons; first the obstruction that parents report most likely represent the apnea and hypopnea events significant for OSA, and second, choking and gasping during sleep is a distinct sound that may be definitively distinguished from that of snoring. In this study, obstruction represents the variable with the strongest correlation to AHI and thus remains in our revised screening tool.
STOP-Bang: The P in our screening tool represents neuropsychological-behavioral symptoms in which excessive tiredness and irritability during the daytime was combined with daytime neurobehavioral symptoms. Positive scores of neurobehavioral symptoms required a diagnosis from a medical professional of either ADHD, ADD, or ODD. Daytime hyperactivity and inattention have been shown to be associated with restless sleep and improved sleep patterns have led to positive changes in behavior.19,36,37 Relationships between OSA, hyperactivity, and inattentive behavior have been documented.19,38–43 Yet excessive tiredness, irritability, and hyperactivity are widely prevalent in children without OSA.19,35,44–48 In this retrospective chart review, parents completed the sleep questionnaire for the majority of subjects under the age of 12, their subjective answers were naturally influenced by their own thoughts, feelings, and attitudes on tiredness and their child’s irritability. The results of this study indicated no relationship to AHI score and the reporting of excessive tiredness/ irritability (p > 0.3). Literature on neurobehavioral symptoms exemplifies a wide range of results. In a study by O’Brien et al., 26% of children with mild symptoms of ADHD were shown to have OSA via a polysomnograph.11,49 A more recent study found that in children 6 to 14 years old with ADHD, OSA was not a common underlying disorder or etiologic factor.11,50 Yet there is evidence to show persistent sleep disturbance can affect cognition, mood, behavior, and family function.11,51 As mentioned previously, the CHAT study ascertained that surgical treatment for OSA in school-age children did not improve attention or executive function.31 Canto’s review showed attention deficit hyperactivity disorder to have a low sensitivity = 52% and a specificity = 67%.26 Based on the lack of evidence that psychological-behavioral symptoms and reports of tiredness/ irritability have significant predictive value, this variable will be omitted in the revised scale.
STOP-Bang: The B in this retrospective chart review denotes body mass index (BMI) percentile for a given age. BMI ≥ 95% indicates an obese child. BMI ≥ 85% to 94% represents children who are overweight. Underweight children are in the BMI ≤ 5% category.52 It was proposed that BMI percentiles > 95% would place a patient at risk for OSA. Obesity has been found to predispose patients to OSA due to the mass loading of upper airway and respiratory muscles, in addition to impairment of ventilation. OSA in obese children ranges from 13% to 36%, based on the severity of obesity.53,54 In our study not only are patients above the 85th percentile at risk for OSA, but also patients below the 10th percentile for BMI. So, using these revised cutoffs, BMI-by-age percentile will remain in the revised screening tool.
STOP-Bang: In our study, age presents the A in STOP-Bang and was defined as a risk factor for patients younger than 3 or older than 13. Evidence of systematic variability with age in pediatric OSA is lacking.55 Our original age parameters are based on the theory that children younger than 3 may have underdeveloped airways, and patients older than 13 are nearing their full growth potential and may start to develop adult risk factors for OSA such as obesity and high blood pressure. After analysis of the data, a reconsideration of age cutoffs is proposed, as it appears in this study that children younger than 4 and older than 16 are at most risk for OSA, yielding specificity as high as 88% and sensitivity as high as 61%. Thus these changes are taken into account in our revised scale.
STOP-Bang: The N in the modified STOP-Bang screening tool represents neuromuscular disorders related to abnormalities of muscle tone, hypotonia, and spasticity influence a child to have OSA.7 The results of this study show a weakly positive relationship to AHI (p = 0.15). This study was limited in that there was a very low sample size of patients who had a neuromuscular disorder (only 21 of 153). This low sample size may have prevented a predictive value with AHI. Neuromuscular deficits, along with craniofacial abnormalities and soft tissue hypertrophy, are frequently the origin of airway narrowing.55 Although neuromuscular disorders did not show a strong correlation to AHI in this study, it remains in the revised scale as it is cited as one of the main causes of OSA.27,29
STOP-Bang: The G in the modified STOP-Bang represents genetic disorders and congenital disorders and like neuromuscular disorders, did not show a strong correlation to AHI in the current study (p = 0.06). Many of these disorders are the underlying etiology of upper airway obstruction as a result of craniofacial malformation.57,58 In the systematic review and meta-analysis by Canto, micrognathia/retrognathia had a sensitivity = 0% with a specificity = 95%. Furthermore, midface hypoplasia overall had a sensitivity = 16% and a specificity = 100%.26 These results ascertain that craniofacial anomalies are not highly predictive of pediatric OSA. Like neuromuscular disorder patients, in our study there was a very small sample size from the data collected—only 21 of 153 subjects had a genetic/congenital disorder. We propose to keep genetic/congenital disorders in the screening tool, as craniofacial anomalies and syndromes were ascertained to be a cause of OSA.27
If the seven revised components mentioned above were used to score the likelihood of OSA, an exploratory multiple logistic regression indicates that more components would have been statistically significant (see Table 4). Even though the p values in the table are not entirely fair, as they are the result of post hoc data mining, it does suggest that the additional factors of BMI risk, age risk, and instances of neuromuscular disorders or genetic/congenital disorders may be important indicators of higher OSA risk. This is consistent with the known subset of children who have the highest risk for OSA including those with underlying abnormalities, such as craniofacial disorders; Down syndrome; cerebral palsy; neuromuscular disorders; chronic lung disease; sickle cell disease; genetic, metabolic, and storage diseases; and laryngomalacia.23
Table 4Revised instrument. |
There were several limitations in this retrospective chart review. The collection of data from the sleep questionnaire proved challenging because many of the sleep questionnaires had inconsistent answers recorded. There remained a lack of verification from the parents reporting and it was not clear whether the patient or parent had filled out the questionnaire. Expectation bias most certainly may have existed in this study, as the examiner was recording the PSG results and also the presence of specific variables that suggest OSA. Prior to 2011, all sleep questionnaires and patient information was recorded in paper charts. These charts were unable to be accessed at the time of data collection; as a result, the sample size was less than originally projected. The sample size does not represent an average population of children as all patients were believed to have a sleep disorder problem and as a result were seeking a diagnosis; thus this study may contain selection bias. Lastly, because this study was retrospective, researchers were limited in what variables could be used in the screening tool as to what information had been previously collected.
There were several variables that this study did not focus on but may be relevant to pediatric OSA. It has been frequently mentioned that there is a genetic component to children with OSA. Future studies may want to include evaluation of whether the parents or siblings currently have a sleep disorder. The siblings of children who have been treated for sleep disorders are more likely have sleep disordered breathing.9,59 In addition, children with a family history of OSA are four times more likely to have OSA than children from families with no OSA diagnosis.60,61 It is also recommended to define snoring both quantitatively and qualitatively to not score children whose snoring is infrequent and not really suggestive of OSA. Mouth breathing during the daytime (sensitivity = 26%, specificity = 79%) and during sleep (sensitivity = 68%, specificity = 42%) was evaluated for diagnostic quality in a previous study.26 Despite the mediocre results, mouth breathing is easily diagnosed by dentists and may be a variable useful for screening patients. Ethnicity may also play a role in screening at risk pediatric patients for OSA. Literature cites that being African American is a risk factor11,62–64; however, this was not found in our study. Kheirandish-Gozal et al. found that the prevalence of OSA was increased in poorly controlled asthmatic children56; perhaps this variable should be included in future studies. Worthy of attention would be a prospective study in which variables typical of pediatric OSA and commonly diagnosed clinically by dentists could be evaluated to determine a predictive value. Further evaluation is recommended to continue to strive and find a highly predictive screening tool for pediatric OSA.
Polysomnography studies have proven labor intensive and have low availability for children. Moreover, Gozal ascertains that “development of simple, cheap, and reliable diagnostic tools that permit more expanded screening of at-risk populations, and enable accurate identification of the children with definitive disease or with definitive absence of disease would revolutionize the field and provide timely access to clinical care to a large sector of the pediatric population, thereby reducing the health burden of OSA.”25 This study attempted to further clarify which variables were strongly associated with childhood OSA, and thus could be used to develop a screening tool that would accurately predict the disorder in at risk children.
CONCLUSIONS
The purpose of the study was to develop a concise and easy-to-use questionnaire as a screening tool to aid in the recognition of OSA in pediatric patients. The screening scale proposed (PM-STOP-Bang) proved to be predictive of pediatric OSA. Based on the results of this study and the review of the literature the following components are recommended to remain in a revised screening tool: presence of snoring, sleep obstruction, tonsillar hypertrophy; BMI, age, neuromuscular disorders and genetic/congenital disorders. Worthy of attention would be to explore ethnicity factors, presence of asthma, and family history of OSA in future studies.
REFERENCES
2. Goroza E, Sagy M, Sagy N, Bock K. Severity assessment of obstructive sleep apnea syndrome (OSAS) in pediatric patients. Clin Pediatr 2009;48:528–33.
3. Dement WC, Vaughan CC. The promise of sleep: a pioneer in sleep medicine explores the vital connection between health, happiness, and a good night’s sleep. New York: Delacorte Press, 1999.
4. Kripke DF, Ancoli-Israel S, Klauber MR, Wingard DL, Mason WJ, Mullaney DJ. Prevalence of sleep-disordered breathing in ages 40–64 years: a population-based survey. Sleep 1997;20:65–76.
5. Levendowski DJ, Morgan T, Montague J, Melzer V, Berka C, Westbrook PR. Prevalence of probable obstructive sleep apnea risk and severity in a population of dental patients. Sleep Breath 2008;12:303–9.
6. Conley RS. Evidence for dental and dental specialty treatment of obstructive sleep apnoea. Part 1: the adult OSA patient and part 2: the paediatric and adolescent patient. J Oral Rehabil 2011;38:136–56.
7. Brown K. Pediatric considerations in sedation for patients with the obstructive sleep apnea syndrome. Semin Anesth 2007;26:94–102.
8. Church G. The role of polysomnography in diagnosing and treating obstructive sleep apnea in pediatric patients. Curr Prob Pediatr Adolesc Health Care 2012;42:2–25.
9. Friedman NR. Novel methods to diagnose obstructive sleep apnea in children. Operative Techniques in Otolaryngoly 2012;23:79–83.
10. Faruqui F, Khubchandani J, Price J, Bolyard D, Reddy R. Sleep disorders in children: a national assessment of primary care pediatrician practices and perceptions. Pediatrics 2011;128:539–46.
11. Ivanhoe J, Lefebvre C, Stockstill J. Sleep disordered breathing in infants and children: a review of the literature. Pediatr Dent 2007;29:193–200.
12. McColley SA, April MM, Carroll JL, Naclerio RM, Loughlin GM. Respiratory compromise after adenotonsillectomy in children with obstructive sleep apnea. Arch Otolaryngol Head Neck Surg 1992;118:940–3.
13. Francis A, Eltaki K, Bash T, Cortes S, Mojdehi K, Goldstein NA. The safety of preoperative sedation in children with sleep-disordered breathing. Int J Pediatr Otorhinolaryngol 2006;70:1517–21.
14. Spruyt K, Gozal D. Pediatric sleep questionnaires as diagnostic or epidemiological tools: a review of currently available instruments. Sleep Med Rev 2011;15:19–32.
15. Chung F, Yegneswaran B, Liao P, et al. STOP questionnaire: a tool to screen patients for obstructive sleep apnea. Anesthesiology 2008;108:812–21.
16. Chung F, Yegneswaran B, Liao P, et al. Validation of the berlin questionnaire and American Society of Anesthesiologists Checklist as screening tools for obstructive sleep apnea in surgical patients. Anesthesiology 2008;108:822–30.
17. Bruni O, Ottaviano S, Guidetti V, et al. The sleep disturbance scale for children (SDSC). construction and validation of an instrument to evaluate sleep disturbances in childhood and adolescence. J Sleep Res 1996;5:251–61.
18. Luginbuehl M, Bradley-Klug K, Ferron J, Anderson WM, Benbadis SR. Pediatric sleep disorders: validation of the sleep disorders inventory for students. School Psychol Rev 2008;37:409–31.
19. Capdevila OS, Kheirandish-Gozal L, Dayyat E, Gozal D. Pediatric obstructive sleep apnea: complications, management, and long-term outcomes. Proc Am Thorac Soc 2008;5:274–82.
20. Harris PA, Taylor R, Thielke R, Payne J, Gonzalez N, Conde JG. Research electronic data capture (REDCap)—A metadata-driven methodology and workflow process for providing translational research informatics support. J Biomed Inform 2009;42:377–81.
21. Chan J, Edman JC, Koltai PJ. Obstructive sleep apnea in children. Am Fam Physician 2004;69:1147–55.
22. Marcus C, Omlin K, Basinki D, Bailey S, Rachal A, Von Pechmann W. Normal polysomnographic values for children and adolescents. Am Rev Respir Dis 1992;146:1235–9.
23. Section on Pediatric Pulmonology, Subcommittee on Obstructive Sleep Apnea Syndrome. American Academy of Pediatrics. Clinical practice guideline: diagnosis and management of childhood obstructive sleep apnea syndrome. Pediatrics 2002;109:704–12.
24. Nieminen P, Tolonen U, Löppönen H. Snoring and obstructive sleep apnea in children: a 6-month follow-up study. Arch Otolaryngol Head Neck 2000;126:481–6.
25. Gozal D. Serum, urine, and breath-related biomarkers in the diagnosis of obstructive sleep apnea in children: is it for real? Curr Opin Pulm Med 2012;18:561–7.
26. Canto GDL, Singh V, Major MP, et al. Diagnostic capability of questionnaires and clinical examinations to assess sleep-disordered breathing in children: a systematic review and meta-analysis. J Am Dent Assoc 2014;145:165–78.
27. Padmanabhan V, Kavitha PR, Hegde AM. Sleep disordered breathing in children--a review and the role of a pediatric dentist. J Clin Pediatr Dent 2010;35:15–21.
28. Hultcrantz E, Löfstrand-Tideström B, Ahlquist-Rastad J. The epidemiology of sleep related breathing disorder in children. Int J Pediatr Otorhinolaryngol 1995;32 Suppl:S63–6.
29. Young T, Peppard PE, Gottlieb DJ. Epidemiology of obstructive sleep apnea: a population health perspective. Am J Respir Crit Care Med 2002;165:1217–39.
30. Costello B, Posnick J. The role of maxillofacial osteotomies in the treatment of obstructive sleep apnea. Curr Opin Otolaryngol Head Neck Surg 2003;11:267–74.
31. Marcus CL, Moore RH, Rosen CL, et al. A randomized trial of adenotonsillectomy for childhood sleep apnea. N Engl J Med 2013;368:2366–76.
32. Bhattacharyya N, Lin HW. Changes and consistencies in the epidemiology of pediatric adenotonsillar surgery, 1996-2006. Otolaryngol Head Neck Surg 2010;143:680–4.
33. Goh DY, Galster P, Marcus CL. Sleep architecture and respiratory disturbances in children with obstructive sleep apnea. Am J Respir Crit Care Med 2000;162:682–6.
34. Fernbach S, Brouillette R, Riggs T, Hunt C. Radiologic evaluation of adenoids and tonsils in children with obstructive sleep apnea: plain films and fluoroscopy. Pediatr Radiol 1983;13:258–65.
35. Melendres MCS, Lutz JM, Rubin ED, Marcus CL. Daytime sleepiness and hyperactivity in children with suspected sleep-disordered breathing. Pediatrics 2004;114:768–75.
36. Minde K, Faucon A, Falkner S. Sleep problems in toddlers: effects of treatment on their daytime behavior. J Am Acad Child Adolesc Psychiatry 1994;33:1114–21.
37. Lavigne JV, Arend R, Rosenbaum D, et al. Sleep and behavior problems among preschoolers. J Dev Behav Pediatr 2000;21:164–9.
38. Chervin RD, Archbold KH. Hyperactivity and polysomnographic findings in children evaluated for sleep-disordered breathing. Sleep 2001;24:313–20.
39. Kaplan BJ, McNicol J, Conte RA, Moghadam HK. Sleep disturbance in preschool-aged hyperactive and nonhyperactive children. Pediatrics 1987;80:839–44.
40. Stein MA, Mendelsohn J, Obermeyer WH, Amromin J, Benca R. Sleep and behavior problems in school-aged children. Pediatrics 2001;107:E60.
41. Chervin RD, Dillon JE, Bassetti C, Ganoczy DA, Pituch KJ. Symptoms of sleep disorders, inattention, and hyperactivity in children. Sleep 1997;20:1185–92.
42. Chervin RD, Archbold KH, Dillon JE, et al. Inattention, hyperactivity, and symptoms of sleep-disordered breathing. Pediatrics 2002;109:449– 56.
43. O’Brien LM, Mervis CB, Holbrook CR, et al. Neurobehavioral implications of habitual snoring in children. Pediatrics 2004;114:44–9.
44. O’Brien LM, Mervis CB, Holbrook CR, et al. Neurobehavioral correlates of sleep-disordered breathing in children. J Sleep Res 2004;13:165–72.
45. O’Brien L, Gozal D. Sleep in children with attention deficit/ hyperactivity disorder. Minerva Pediatr 2004;56:585–601.
46. Beebe DW. Neurobehavioral morbidity associated with disordered breathing during sleep in children: a comprehensive review. Sleep 2006;29:1115–34.
47. Gottlieb DJ, Vezina RM, Chase C, et al. Symptoms of sleep-disordered breathing in 5-year-old children are associated with sleepiness and problem behaviors. Pediatrics 2003;112:870–7.
48. Montgomery-Downs H, Jones VF, Molfese VJ, Gozal D. Snoring in preschoolers: associations with sleepiness, ethnicity, and learning. Clin Pediatr 2003;42:719–26.
49. O’Brien LM, Holbrook CR, Mervis CB, et al. Sleep and neurobehavioral characteristics of 5- to 7-year-old children with parentally reported symptoms of attention-deficit/hyperactivity disorder. Pediatrics 2003;111:554–63.
50. Sangal RB, Owens JA, Sangal J. Patients with attention-deficit/ hyperactivity disorder without observed apneic episodes in sleep or daytime sleepiness have normal sleep on polysomnography. Sleep 2005;28:1143–8.
51. Stores G. Sleep-wake function in children with neurodevelopmental and psychiatric disorders. Semin Pediatr Neurol 2001;8:188–97.
52. Tseng R, Vann WF, Perrin EM. Addressing childhood overweight and obesity in the dental office: rationale and practical guidelines. Pediatr Dent 2010;32:417–23.
53. Ng DK, Chow P, Chan C, Kwok K, Cheung JM, Kong FY. An update on childhood snoring. Acta Paediatr 2006;95:1029–35.
54. Ng D, Lam Y, Kwok K, Chow P. Obstructive sleep apnea syndrome and obesity in children. Hong Kong Med J 2004;10:44–8.
55. Katz ES, D’Ambrosio CM. Pediatric obstructive sleep apnea syndrome. Clin Chest Med 2010;31:221–34.
56. Kheirandish Gozal L, Dayyat E, Eid N, Morton R, Gozal D. Obstructive sleep apnea in poorly controlled asthmatic children: effect of adenotonsillectomy. Pediatr Pulmonol 2011;46:913–8.
57. Miloro M. Mandibular distraction osteogenesis for pediatric airway management. J Oral Maxillofac Surg 2010;68:1512–23.
58. Cohen SR, Simms C, Burstein FD. Mandibular distraction osteogenesis in the treatment of upper airway obstruction in children with craniofacial deformities. Plast Reconstr Surg 1998;101:312–8.
59. Friberg D, Sundquist J, Li X, Hemminki K, Sundquist K. Sibling risk of pediatric obstructive sleep apnea syndrome and adenotonsillar hypertrophy. Sleep 2009;32:1077–83.
60. Kaditis A, Kheirandish Gozal L, Gozal D. Algorithm for the diagnosis and treatment of pediatric OSA: a proposal of two pediatric sleep centers. Sleep Med 2012;13:217–27.
61. Redline S, Tishler PV, Schluchter M, Aylor J, Clark K, Graham G. Risk factors for sleep-disordered breathing in children. associations with obesity, race, and respiratory problems. Am J Respir Crit Care Med 1999;159:1527–32.
62. Harding SM. Prediction formulae for sleep-disordered breathing. Curr Opin Pulm Med 2001;7:381–5.
63. Redline S, Amin R, Beebe D, et al. The childhood adenotonsillectomy trial (CHAT): rationale, design, and challenges of a randomized controlled trial evaluating a standard surgical procedure in a pediatric population. Sleep 2011;34:1509–17.
64. Rosen CL, Larkin EK, Kirchner HL, et al. Prevalence and risk factors for sleep-disordered breathing in 8- to 11-year-old children: association with race and prematurity. J Pediatr 2003;142:383–9.
SUBMISSION & CORRESPONDENCE INFORMATION
Submitted for publication May, 2015
Submitted in final revised form June, 2015
Accepted for publication June, 2015
Address correspondence to: Harmeet K. Chiang, PO Box 566, School of Dentistry, Virginia Commonwealth University, Richmond, VA 23298- 0566; Email: hkchiang@vcu.edu
DISCLOSURE STATEMENT
This was not an industry supported study. The authors have indicated no financial conflicts of interest.
APPENDIXES
Appendix 1Data sheet. |
Appendix 2VCU Center for Sleep Medicine Questionnaire. |