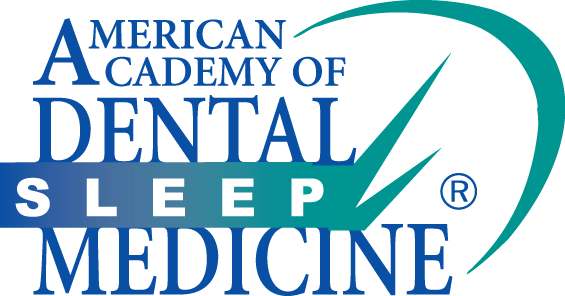
Original Article 1, Issue 2.4
How Close Can Single-Channel EMG Data Come to PSG Scoring of Rhythmic Masticatory Muscle Activity?
http://dx.doi.org/10.15331/jdsm.5114
Patricia Dreyer, DDS1 ; Wataru Yachida, DDS, PhD2 ; Nelly Huynh, PhD3 ; Gilles J. Lavigne, DMD, PhD3 ; Morten Haugland, MSc, PhD4 ; Peter Svensson, DDS, PhD, Dr Odont1,5,6; Eduardo E. Castrillon, DDS, MSc, PhD1,6
1 Section of Orofacial Pain and Jaw Function, Department of Dentistry, HEALTH, Aarhus University, Aarhus C, Denmark; 2Department of Oral Rehabilitation, Graduate School of Dental Medicine, Hokkaido University, Sapporo, Japan; 3Faculté de Médecine Dentaire, Université de Montréal, Montréal, QC, Canada; 4R&D Engineer at DELTA, Hørsholm, Denmark; 5Karolinska Institute, Department of Dental Medicine, Huddinge, Sweden, 6Scandinavian Center for Orofacial Neuroscience (SCON)
ABSTRACT
Study Objectives:
Assessment of jaw-muscle activity during sleep is needed to establish a definite diagnosis of sleep bruxism (SB). Multichannel polysomnographic (PSG) studies are the gold standard (GS) but are unfortunately not readily available, so single-channel electromyographic (EMG) devices have been developed. This study attempted to evaluate an EMG algorithm for single-channel EMG recordings in comparison with the outcome from PSG recordings.
Methods:
PSG data from 20 participants with different frequency of jaw-muscle EMG activity were analyzed with the GS algorithm, including previously published criteria for EMG analyses and contrasted to two different algorithms: one based on a signal recognition (SR) algorithm and the other based on a moving average (MA) estimation method, which is characterized by a comparison of the EMG amplitude to the estimated background level, and applying the rules for detection of rhythmic masticatory muscle activity (RMMA).
Results:
The highest correlation coefficients (r = 0.96) were obtained between the GS and the MA algorithm; however, there were no significant differences in the absolute numbers of EMG bursts or episodes between the SR and MA algorithms and GS during sleep. However, both algorithms significantly overestimated the EMG bursts and episodes when awakenings during sleep were included in the analyses. There were no significant differences between muscles or side (p > 0.06).
Conclusions:
This study strongly indicates that a MA algorithm may be useful for analysis of EMG activity during sleep but with recognition of the potential overestimation of EMG bursts and episodes due to transient awakenings.
Keywords:
sleep bruxism, polysomnography, single-channel electromyographic device, rhythmic masticatory muscle activity, electromyography
Citation:
Dreyer P, Yachida W, Huynh N, Lavigne GJ, Haugland M, Svensson P, Castrillon EE. How close can single-channel EMG data come to PSG scoring of rhythmic masticatory muscle activity? Journal of Dental Sleep Medicine 2015;2(4):147–156.
Bruxism is a repetitive jaw-muscle activity characterized by clenching or grinding of the teeth and/or by bracing or thrusting of the mandible that could happen during awake and sleep periods.1 Although sleep bruxism (SB) is not a life-threatening disorder, it can affect the patient’s quality of life, especially because of dental problems such as tooth wear, damage or fractures of tooth structures or dental restorations, pain in the orofacial region, and tension-type headache.2,3 SB is often suggested as a cause of temporomandibular disorders (TMD) orofacial pain and headache, but scientific evidence does not support a simple link between SB and craniofacial pain conditions.4–7 A set of clinical research diagnostic criteria (RDC) for SB were proposed in 19968 and has since been considered the gold standard (GS) in many clinical studies of SB. The criteria were re-validated by Rompré et al.9 and used for classification of a subgroup of bruxism patients with a higher risk of pain. Still, the relation between SB and many adverse clinical symptoms and signs is unclear, and the factors causing SB and the physiological mechanisms behind SB are still being discussed.3
One reason for the relative paucity of research data on SB may be that the RDC includes use of full polysomnographic (PSG) and audio-video recordings that can be done in sleep laboratory or at home (type 1 and 2 type recording system, respectively). While PSG and audio-video recordings provide highly accurate scoring of rhythmic masticatory muscle activity (RMMA),8 it is also a costly and time-consuming procedure, which is difficult to do for most clinicians and even for most research groups. It also requires training of the person scoring the data, and still there is some level of discrepancy between how the data are scored by different scorers and from different laboratories.10
Due to the costs and efforts associated with PSG, there has been great interest in scoring RMMA with more simple and portable devices that can be used over long periods of time in the patients’ own home.11–18 These recording systems can be type 3 (with 3–4 physiological variables such as muscle, cardiac, and respiration) or simplified type 4 with one EMG channel. Recently, a portable single-channel EMG device was introduced for recordings of jaw-muscle activity during sleep based on a signal recognition (SR) algorithm.15,16 However, single-channel EMG devices are notoriously known to record too many “true” events of RMMA,18 and the absence of audio-video also complicate the scoring, resulting in an overestimation of 25%.19
To overcome this problem, the original Grindcare SR algorithm was updated. The modified algorithm is characterized by a comparison of the EMG amplitude to the estimated background level (moving average, MA) and applying the rules for detection of RMMA activity described by Lavigne and collaborators8 for quantification of RMMA.
Thus, the overall aim of the present study was to determine how close a simple algorithm of a single-channel EMG could come to PSG and audio-video recording in terms of quantification of RMMA during sleep. Specifically, there were three main objectives: (1) investigate the performance of two signal analysis methods, the SR and the MA vs. GS (RMMA); (2) investigate if there was a significant difference in the quantification of RMMA from EMG activity recorded from masseter or temporalis muscles or between left/right body sides; and (3) determine the impact of awake periods before, during, or after sleep on the quantification of RMMA frequency.
MATERIALS AND METHODS
Subjects
Data from a total of 20 individuals (12 women/8 men) were selected for this retrospective analysis (mean age: 26.8 ± 1.41 years). The data used in the present study had all been recorded previously, as part of a standard PSG examination at University of Montreal and this accordingly to ethical standards of Sacre Coeur Hospital research center. Six datasets were selected from healthy control subjects with no history or physical signs of bruxism. The other 14 sets were patients with a positive history and physical signs of bruxism but otherwise no neurological or sleep disorders.9,19,20 This combination of individuals with and without history and physical signs of bruxism was chosen to reflect the continuum of RMMA during sleep, i.e., to avoid ceiling or floor effects in the detection of RMMA with the different EMG algorithms.
Polysomnographic Data
The PSG data included electroencephalography (EEG: 7 channels), electrooculography (EOG: 2 channels), EMG from the masticatory muscles (right anterior temporalis muscle TAR, left anterior temporalis muscle TAL, right masseter muscle MAR, and left masseter muscle MAL), EMG from the leg muscles (anterior tibialis muscle), electrocardiogram (ECG: 3 channels), audio and video recordings. EMG data was low-pass filtered at 70 Hz and sampled at a 256 samples/ second rate.The PSG data from all 20 individuals had been scored for sleep stage and for RMMA activity according to standard and published routines at University of Montreal.8,9 This scoring was done manually, based on all the available information described above, as well as audio and video recordings. The compiled information from the PSG scoring (“RMMA burst” and “RMMA episodes”) was considered to be the GS in the present study and was provided in addition to the raw EMG data. Data was translated from Steallate (Natus) format and saved in the European Data Format for Biosignals (EDF). This enabled us to read the signals into Matlab (Mathworks Inc) for analysis. The GS information on RMMA was then compared to the outcome from 2 different EMG algorithms (SR; MA), which were applied to the 4 masticatory EMG channels. The PSG data from all the 20 individuals were scored and an “RMMA index” was calculated, which is the total number of RMMA episodes activity divided by sleep duration.
Due to the fact that it is normal to have transient awakening periods during sleep the data was also classified as EMG activity during sleep (“sleep period”) and EMG activity during sleep plus awakenings (“sleep + awakenings period”). The GS criteria to score RMMA excluded the transient awaken periods using the sleep staging.
EMG Algorithms
Signal Recognition Algorithm
The first EMG algorithm was an approximation of the SR algorithm used in the original Grindcare device (Medotech A/S, Herlev, Denmark).15 This algorithm was developed to work along with contingent electrical stimulation, which imposes some constraints in terms of EMG measurement and stimulation through the same electrode. Very briefly, this EMG algorithm compares the amplitude of the EMG to a threshold level, which is set to 20% of the maximum EMG during a clench to about 60% of the maximum voluntary contraction (MVC). Setup of the threshold level is done every time the device is mounted before sleep, during which the user is required to produce a bite force to approximately 60% MVC. An “SR grind” is detected, counted in the log-file and registered when the amplitude of the EMG signal has been above the threshold for more than 0.1 s.For the purpose of the contingent electrical stimulation, it was thought to be important that the electrical stimulation was delivered as soon as possible after detection of the EMG activity. This means that it is not possible for the device to wait and see if an EMG burst is indeed part of an episode of rhythmic EMG activity, a long (tonic) EMG burst, or merely a single brief EMG event. The electrical stimulation is delivered as soon as any EMG activity is detected, and for the next 1 second, the stimulation interferes with the EMG recording. This means it is not possible to measure the duration of bursts of EMG activity and counting of bursts is difficult, due to the 1-second “blind period.” When 1 second has passed, the EMG signal is monitored again, and if above threshold, a new EMG event can be detected. This has the implication that if a long burst of EMG appears in the signal (i.e., > 1 second), several events may be detected and counted.
It was, however, not possible to do a full simulation of the Grindcare SR algorithm due to differences in filtering and sample rate between standard PSG equipment and Grindcare. Grindcare samples at a much higher rate (2,000 samples/s compared to 256 samples/sec) and removes the low frequency content in the signal, in order to reduce possible interference of low-frequency noise (e.g., 50/60 Hz noise). Moreover, Grindcare uses a fast Fourier transform-based, proprietary, method for further reducing the influence of noise and detection of pure EMG signals. This part of the EMG analysis was not possible to simulate with the available PSG data. However, in the sleep laboratory measures have been taken to reduce interfering noise, and operators continuously ensure that there is good electrode contact and that the signals look good, reducing the need for the noise reduction techniques used with Grindcare. Unfortunately, the datasets provided from the sleep laboratory did not include EMG data, wherein the subject was instructed to clench to 60% MVC. There was, however, a part of the standard “biocalibration,” where individuals were instructed to “clench teeth strongly together 3 times.”
Moving Average Algorithm
This MA algorithm for detection of EMG bursts used a dynamic method for estimation of background EMG noise. Bursts of EMG that exceeded the background noise with more than 3 times the background amplitude were detected. Furthermore, the rules for classification of EMG as RMMA as described in Lavigne et al.8 were applied: An EMG burst must be ≥ 0.25 sec in duration, an EMG burst can be phasic (< 2 sec) or tonic (> 2 sec), and an EMG burst must be part of an EMG episode to be counted; an EMG episode consists of either ≥ 3 phasic EMG bursts and/or one or more tonic EMG bursts; an EMG episode consists of EMG bursts < 3 sec apart. The EMG bursts and episodes detected using this MA algorithm will be called “MA burst” and “MA episode.”Both the SR and MA algorithms were applied to the EMG recordings from MAL, MAR, TAL, TAR in all 20 individuals. Analyses were done by Morten Haugland in Denmark blind to SB diagnostic or RMMA episodes frequency of data collected in Montreal.
Statistics
Kolmogorov-Smirnov tests indicated the majority of parameters were not normally distributed, and therefore nonparametric descriptive statistics (median and interquartile ranges) were applied except for the RMMA index. Friedman repeated measures analysis of variance on ranks (ANOVA) was used to test the EMG data. We compared the number of SR grinds, MA bursts, and RMMA bursts per hour of sleep. Moreover, MA episodes and RMMA episodes (GS) were also compared. PSG data were also compared between algorithms (3 levels: SR, MA GS), masticatory muscles (4 levels: MAL, MAR, TAL, TAR) and between PSG sleep EMG or sleep + awakening EMG (2 levels). Tukey post hoc tests were used to compensate for multiple comparisons and the Dunnett method when appropriate. The RMMA-index was compared between bruxers and non-bruxers with the use of an unpaired t-test. Pearson product moment correlation tests were used to test for associations between the outcomes from the 2 algorithms versus the GS criteria. This was done for all EMG channels (MAL, MAR, TAL, TAR) and for PSG data containing both sleep and sleep including awakening. P < 0.05 was considered statistically significant. Moreover, levels of agreement between outcomes from the 2 algorithms versus GS criteria were tested using Bland-Altman test.RESULTS
Polysomnographic Data
Figure 1 shows an example of a full-night PSG recording with focus on the right masseter muscle. The awaken periods have been excluded with the use of the sleep staging and the analyses of the EMG activity shown according to the GS criteria, SR and MA algorithms. From this example it is evident that the SR algorithm due to its inherent nature overestimates the number of SR grinds compared to the number of MA episodes detected by the MA algorithm and the RMMA episodes of the GS.
Figure 1Illustration of PSG data from a single subject (#4). |
The GS scoring showed that the RMMA index for the included data ranged from 0.3 episodes/h of sleep to 13.5 episodes/h of sleep (Table 1). The mean RMMA index in the designated sleep bruxers was 4.9 ± 3.5 episodes per hour of sleep compared to 1.4 ± 0.8 episodes per hour of sleep in nonbruxers (unpaired t-test: p = 0.03).
Table 1Characteristics of PSG data from 20 individuals. |
Comparison between Algorithms
The ANOVA indicated no significant difference between the number of SR grinds, MA bursts and RMMA bursts detected with the SR, MA algorithms, and the GS during sleep period (p > 0.40, Figure 2A). However, both algorithm SR and MA detected significantly more SR grinds/MA bursts than the RMMA bursts of GS during sleep + awakening period (p < 0.02, Tukey: p < 0.05, Figure 2B).
Figure 2Comparison between algorithms. |
The ANOVA for episodes did not include the number of SR algorithm analyses results but only contrasted the MA episodes and the RMMA episodes of GS: There were no significant differences on the episodes between these 2 algorithms (p = 0.19, Figure 3A) during sleep, but significantly higher MA episodes during sleep + awakenings period (p < 0.001, Tukey: p < 0.05, Figure 3B).
Figure 3Comparison between algorithms. |
Comparison between Muscles
There were no significant differences on the number of SR grinds/MA bursts and on the number of MA episodes detected between the 4 masticatory muscles (p > 0.06) (Figure 2 and 3).Comparison between Sleep and Sleep Including Awakening
The quantitative analyses clearly indicated that the number of SR grinds, MA bursts (Figure 2), and MA episodes (Figure 3) during the sleep period were significantly lower than the during sleep + awakening period (p < 0.001).Correlations and Levels of Agreement between Different Algorithms
Figure 4 shows the correlation plots between the RMMA (GS) bursts and SR grinds using the GS criteria and SR algorithm for the 4 masticatory muscles recorded only during sleep. In a similar way, Figure 5 and 6 shows the plots of the data of RMMA and MA algorithm for the four different muscles recorded only during sleep (bursts and episodes, respectively; Table 2, 3).
Figure 4Correlations and levels of agreement between different algorithms. |
Figure 5Correlations and levels of agreement between different algorithms. |
Figure 6Correlations and levels of agreement between different algorithms. |
Table 2Correlation of SR and MA outcomes with the RMMA. |
Table 3Correlation of MA episodes with RMMA episodes. |
Moreover, levels of agreement between outcomes from the 2 algorithms versus GS criteria are shown in Table 4.
Table 4Bland Altman test results of the agreement between SR/MA and GS assessment methods. |
DISCUSSION
The Grindcare device has, until now, scored muscle activity using SR algorithm, as defined above.15 The proposed MA algorithm uses a novel adaptive threshold to determine the occurrence of MA bursts and the rules for detection of bursts and episodes activity were applied in a similar way as when RMMA is scored manually following GS criteria. The algorithms were compared with correlation coefficients and differences between the total amounts of EMG activity detected with the different methods.
Bursts and episodes detected with the MA algorithm in general correlated well with the manual scoring based on the full PSG data. The datasets scored in PSG analysis as having “high” RMMA frequency, in most cases also came out as being highest when scored on a single channel of EMG. However, it was more difficult to distinguish between datasets with “mild” RMMA from the control subjects and the patients with normal RMMA frequency.
For comparison, the algorithm of another commercial device, Bitestrip, is described in brief below. The Bitestrip is a single-use device, to be placed on the masseter muscle. It measures and quantifies activity in a way that is rather similar to the SR algorithm.13 The threshold is set at 30% of maximal voluntary clenches. Thirty minutes following activation (to allow time for falling asleep), the device begins counting continuously throughout the recording period those EMG masseter events that are at or above threshold for more than 0.25 s. A single count is limited to 1 s, thus an event > 1 s is counted as an additional event, as long as the additional time following the event already counted exceeds 0.25 s. The Bitestrip device classifies the results into 4 classes: L = Very low sleep bruxism (0–30 events), 1 = Mild sleep bruxism (31–60 events), 2 = Moderate sleep bruxism (61–100 events), 3 = Severe sleep bruxism (> 101 events). Only the class is given as output to the user. The SR and MA algorithms provide a numerical output report facilitating the interpretation of the assessment of continuous multiple nights EMG recordings. This technique allows the study of other aspects such us variability, tendency, long-term averages, etc. Even though the SR algorithm did not show significant correlations with GS (Figure 4, Table 2), the ANOVA indicated no significant difference between the number of SR grinds, MA bursts and RMMA bursts detected with the SR and MA algorithms and the GS during sleep (p > 0.40, Figure 2A).
Although the manual scoring of RMMA is based on the complete set of data from the PSG recording, the EMG from the right masseter is used to determine the precise timing of bursts of activity. The rest of the information is used to determine whether the activity is true RMMA or other orofacial activity/noise. For long-term home-use of a single-channel EMG device, the anterior temporalis muscle is a more convenient choice in most patients, as it is more practical to have an electrode placed on the temple rather than on the cheek, especially for people with a beard.21 Moreover, there seems to be no practical difference in using EMG from either the masseter or the temporalis muscle, and there was no practical difference in using either side of the head. This is in accordance with the bilateral although not symmetrical motor control of the jaw-closing muscles.22
The new MA algorithm had a good correlation with the PSG scoring, when looking at periods of sleep only. However, only 77% of the RMMA episodes found by PSG analysis coincided with the MA episodes found by the MA algorithm, and only 64% of the bursts. If considering only the overall number of bursts and episodes, there seems to be no practical difference in using the number of bursts or number of episodes of RMMA for classification purposes. However, the better match between individual episodes scored implies that the number of episodes is a more robust measure.
Inclusion of awake periods in the analysis reduced the correlation between the single-channel EMG methods and the PSG scoring and generally increased the number of bursts/ hour. The correlation was still fair, and the ordering of RMMA frequency was still reasonable. It is needed to highlight that the GS criteria to score RMMA excludes the transient awaken periods using the sleep staging. This exclusion can be made because the full PSG recordings including audio and video recordings make it possible to discriminate these transient awaken periods.
The present results shall be seen as a preliminary study, using previously recorded data only, to indicate to which extent a single EMG-channel can be used for scoring RMMA. Further, the knowledge gained in the study will be used for guiding the implementation of RMMA detection algorithms in a singlechannel ambulatory device.
A single-channel EMG device is, however, limited in terms of diagnosis, because it is considered a type 4 device according to AASM, i.e., a screening tool under clinical use. Even so, the advantages of being able to collect large amounts of data, from many subjects, over long periods of time, in their own homes, may outweigh at least some of the limitations, especially if the device is commercially available, easy to use, and provides detailed information that is related to that provided by PSG, while the limitations are known and well described.
In light of the present results we can conclude: (1) No significant difference was observed between the number of SR grinds, MA bursts, and RMMA bursts detected with the SR and MA algorithms and the GS during sleep. Therefore SR grinds and MA bursts may be useful for analyses of EMG activity during sleep and comparable to the GS. Nevertheless, we have to take into account that both the SR and MA algorithms detected significantly more grinds/bursts than the GS during sleep including awakening. (2) There was no significant difference in the quantification of RMMA based on either the EMG activity in the masseter or temporalis muscles and between sides. (3) The number of SR grinds, MA bursts and RMMA bursts, and MA episodes and RMMA episodes during sleep periods were significantly lower compared with during sleep + awakening periods.
REFERENCES
2. Lavigne GJ, Khoury S, Abe S, Yamaguchi T, Raphael K Bruxism physiology and pathology: an overview for clinicians. J Oral Rehabil 2008;35:476–94.
3. Svensson P, Lavigne GJ. Sleep related bruxism. In: Kushida CA, ed. Encyclopedia of Sleep. San Diego, CA: Academic Press, 2013:5–57.
4. Svensson P, Jadidi F, Arima T, Baad-Hansen L, Sessle BJ. Relationships between craniofacial pain and bruxism. J Oral Rehabil 2008;35:524–47.
5. Manfredini D, Lobbezoo F. Relationship between bruxism and temporomandibular disorders: a systematic review of literature from 1998 to 2008. Oral Surg Oral Med Oral Pathol Oral Radiol Endod 2010;109:e26–50.
6. Raphael KG, Sirois DA, Janal MN, et al. Sleep bruxism and myofascial temporomandibular disorders: a laboratory-based polysomnographic investigation. J Am Dent Assoc 2012;143:1223–31.
7. Yachida W, Castrillon EE, Baad-Hansen L, et al. Craniofacial Pain and jaw-muscle activity during sleep. J Dent Res 2012;91:562–7.
8. Lavigne GJ, Rompre PH, Montplaisir JY Sleep bruxism: validity of clinical research diagnostic criteria in a controlled polysomnographic study. J Dent Res 1996;75:546–52.
9. Rompre PH, Daigle-Landry D, Guitard F, Montplaisir JY, Lavigne GJ. Identification of a sleep bruxism subgroup with a higher risk of pain. J Dent Res 2007;86:837–42.
10. Gallo LM, Lavigne G, Rompre P, Palla S Reliability of scoring EMG orofacial events: polysomnography compared with ambulatory recordings. J Sleep Res 1997;6:259–63.
11. Gallo LM, Guerra PO, Palla S Automatic on-line one-channel recognition of masseter activity. J Dent Res 1998;77:1539–46.
12. Rivera-Morales WC, McCall WD Jr. Reliability of a portable electromyographic unit to measure bruxism. J Prosthet Dent 1995;73:184–9.
13. Shochat T, Gavish A, Arons E, et al. Validation of the BiteStrip screener for sleep bruxism. Oral Surg Oral Med Oral Pathol Oral Radiol Endod 2007;104:e32–9.
14. Mizumori T, Inano S, Sumiya M, Kobayashi Y, Watamoto T, Yatani H. An ambulatory bruxism recording system with sleep-stage analyzing function. J Prosthodont Res 2009;53:150–4.
15. Jadidi F, Castrillon E, Svensson P. Effect of conditioning electrical stimuli on temporalis electromyographic activity during sleep. J Oral Rehabil 2008;35:171–83.
16. Jadidi F, Norregaard O, Baad-Hansen L, Arendt-Nielsen L, Svensson P. Assessment of sleep parameters during contingent electrical stimulation in subjects with jaw muscle activity during sleep: a polysomnographic study. Eur J Oral Sci 2011;119:211–8.
17. Ahlberg K, Savolainen A, Paju S, et al. Bruxism and sleep efficiency measured at home with wireless devices. J Oral Rehabil 2008;35:567–71.
18. Yamaguchi T, Abe S, Rompre PH, Manzini C, Lavigne GJ Comparison of ambulatory and polysomnographic recording of jaw muscle activity during sleep in normal subjects. J Oral Rehabil 2012;39:2–10.
19. Koyano K, Tsukiyama Y, Ichiki R, Kuwata T. Assessment of bruxism in the clinic. J Oral Rehabil 2008;35:495–508.
20. Lavigne GJ, Cistulli PA, Smith MT. Sleep medicine for dentists: a practical overview. Chicago, IL: Quintessence Pub., 2009.
21. Arima T, Tomonaga A, Yachida W, et al. Site-to-site variation of muscle activity and sensitivity in the human anterior temporalis muscle: implications for contingent stimulation. Acta Odontol Scand 2012;70:89–95.
22. Nordstrom MA, Miles TS, Gooden BR, Butler SL, Ridding MC, Thompson PD. Motor cortical control of human masticatory muscles. Prog Brain Res 1999;123:203–14.
SUBMISSION & CORRESPONDENCE INFORMATION
Submitted for publication February, 2014
Submitted in final revised form May, 2015
Accepted for publication August, 2015
Address correspondence to: Eduardo E. Castrillon DDS, MSc, PhD, Section of Orofacial Pain and Jaw Function, Department of Dentistry, HEALTH, Aarhus University, Vennelyst Boulevard 9, DK-8000 Aarhus C, Denmark; Tel.: +(45) 87 16 82 59; Fax: +(45) 86 19 56 65; Email: ec@ odont.au.dk
DISCLOSURE STATEMENT
The study was partially supported by Medotech A/S. M. Haugland worked for the company. Data collection in Montreal, Canada was possible with a CIHR grant to Dr. Lavigne. MH was formerly an employee of Medotech, (previous Grindcare manufacturer) from 2008 to 2012. Dr. Castrillon was partially supported for a post doctorate fellowship at Aarhus University 2009 to 2010 by Medotech. Dr. Svensson was a member of the advisory board of Medotech. Drs. Lavigne and Huynh participated in an advisory board meeting of Medotech, in Montreal in 2010. They also tested a type 3 recorder from Braebon, Canada without any financial link. They have no financial share to any of the companies. Dr. Lavigne is a Canada Research Chair. The other authors have indicated no clonflicts of interest.